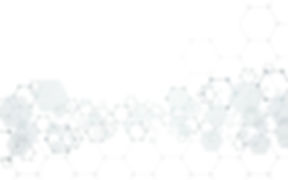
RESEARCH
SysMet: Integrative Systems Metabolomics
Phase I Small Business Innovation Research (SBIR)
Metabolomics plays an indispensable role in the growing systems biology approaches to identify reliable cancer biomarkers. Liquid chromatography coupled to mass spectrometry (LC-MS) and gas chromatography coupled to mass spectrometry (GC-MS) have been extensively used for high-throughput comparison of the levels of thousands of metabolites among biological samples. However, the potential values of many disease-associated analytes discovered by these platforms have been inadequately explored in systems biology research due to lack of computational tools. Partly due to these limitations, poor reproducibility of previously identified metabolite biomarker candidates has been observed, especially when they are evaluated through independent platforms and validation sets. This project aims to address this challenge using a new software tool (SysMet) that utilizes a network-based approach to uncover relationships between disease and metabolites by investigating the rewiring and conserved interactions among metabolites in the progression of the disease. In addition, we propose to extend the network-based approach for integrative analysis of multi-omics data to identify disease-associated metabolites. The tool will contribute to improving the ability of researchers to discover biomarkers by enhancing the role of metabolomics in systems biology research.
MetaboCraft: Tool for Metabolite Identification
Phase I Small Business Innovation Research (SBIR)
In a typical untargeted metabolomics analysis by liquid chromatography-mass spectrometry (LC-MS), about 70% of the detected ions represent unknown analytes. While identification of the unknowns without putative IDs remains a significant challenge, we have the opportunity to identify more metabolites by improving the ability to prioritize multiple putative IDs assigned to the known-unknowns. This will be tremendously helpful in selecting promising metabolites for the subsequent experimental verification of the IDs. This project seeks to develop a probabilistic framework that assigns a priority score to each putative metabolite ID by combining information from multiple resources including compound databases, pathways, biochemical networks, and spectral libraries. The proposed probabilistic model will exploit the inter-dependent relationships between metabolites in biological organisms based on knowledge derived from pathways and biochemical networks to assign priority score to each putative metabolite IDs. If MS/MS data are available, the score for a putative ID will take into account how well the measured MS/MS matches against those in spectral libraries or fragment patterns predicted by in-silico spectral interpretation. Successful implementation and validation of the model will enable users to accurately identify putative metabolite IDs and assign priority scores by taking advantage of publicly available databases, pathways, and biochemical networks, spectral libraries, as well as various tools designed for isotope/adduct recognition, decomposition of isotopic patterns, and in-silico spectral interpretation.
MetaboQuest: Tool for Metabolite Identification
Phase II Small Business Innovation Research (SBIR)
The goal of this Phase II SBIR proposal is to make metabolomics studies on a par with other omics studies such as genomics, transcriptomics, and proteomics, for which well-established pipelines are available. By doing so, we will accelerate the role of metabolomics in systems biology approaches for various applications including biomarker and drug discovery. To achieve this goal, we propose to develop a cloud-based platform that allows customers to build pipelines for analysis of LC-MS-based untargeted metabolomics data, starting from peak detection to metabolite annotation. This will be accomplished by implementing a suite of innovative tools that can be assembled into customized pipelines and by enhancing metabolite annotation accuracy through integration of information derived from multiple resources including compound databases, pathways, biochemical networks, and mass spectral libraries. Successful implementation and validation of MetaboQuest will contribute to addressing the major bottleneck in metabolomics - metabolite identification, thereby eliminating the need for manual verification of putative metabolite IDs and enhancing the contribution of metabolomics studies, specifically in disease biomarker and drug discovery.
iSysMet: A Cloud-Based Pipeline for Integrative Analysis of Multi-Omics and Imaging Data
Phase I Small Business Innovation Research (SBIR)
Recent advances in cloud-based resources and technologies for multi-omics and imaging analysis have created new opportunities for exploring relationships between histology, molecular events, and clinical outcomes using quantitative methods. However, the unprecedented scale and complexity of multi-omics and imaging data have presented critical computational bottlenecks requiring new concepts and enabling tools. The objective of this proposal is to address the computational challenges in integrative analysis of multi-omics and imaging data from The Cancer Genome Atlas (TCGA), Clinical Proteomic Tumor Analysis Consortium (CPTAC), and The Cancer Imaging Archive (TCIA) via an innovative cloud-based data analytics pipeline to fully unlock the potential of the Cancer Research Data Commons (CRDC). This will be accomplished by building a computational framework that integrates novel big data analysis algorithms into a cloud-based pipeline for revealing complex relationships between histopathology images, multi-omics, and phenotypic outcomes. This project not only facilitates the development of new big data analysis techniques, but also addresses emerging scientific questions in cancer research via a cloud-based data analytics pipeline that consists of innovative computational methods for multi-omics and imaging analysis and interfaced with CRDC. The proposed computational methods and pipeline are expected to impact cancer research and enable investigators to effectively test their scientific hypothesis.
MetCraft: Pipeline for Analysis of Metabolomics Data
Phase I Small Business Innovation Research (SBIR)
The broader impact of this Small Business Innovation Research (SBIR) Phase I project is enhancing the role of metabolite (substances used by cells for growth, reproduction and health) analysis in the growing area of systems biology research. This is expected to lead to faster and less expensive biomarker and drug discovery to allow for more accurate, reproducible, and faster clinical trials, and to accelerate basic scientific research into many areas of cellular and system-wide organismal studies. This will have a significant impact on the bottom line for drug companies and for improving health and reducing health care cost. The innovation will provide customers with a platform and expertise that enable them to increase their ability to develop biomarkers and drugs faster by: (1) allowing more metabolites to be involved in the discovery of new relationships between diseases and metabolites, potentially opening up new areas of basic research; (2) selecting disease-associated metabolites on the basis of not only statistically significant changes in metabolite levels but also correlations of interactions among metabolites in diseased vs. healthy cells; and (3) evaluating the relationships between metabolites and diseases through integration of metabolite analysis with other system-wide analytical methods (i.e. gene expression, protein levels, etc.).


FINANCIAL CONFLICTS OF INTEREST POLICY
OMICSCRAFT LLC
Effective Date: January 26, 2022
Policy Statement. OMICSCRAFT is committed to ensure the integrity of its research and to comply with the federal Public Health Service’s (PHS) requirements and regulations (Title 42 Code of Federal Regulations (CFR), Part 50, Subpart F, Responsibility of Applicants for Promoting Objectivity in Research for Which PHS Funding is Sought)) for institutions that seek research funding. OMICSCRAFT’s Financial Conflicts of Interest (FCOI) Policy seeks to identify potential, actual, or apparent financial conflicts of interest, and provides processes for disclosure of FCOIs and eliminating and/or managing them as appropriate. OMICSCRAFT’s Investigators participating in any Research or Research through subgrantees, contractors, or collaborators that are funded by any PHS agency or any non-PHS organization that has adopted the PHS FCOI Regulations are required to comply with this Policy. This Policy is effective as of January 26, 2022 and will be updated annually, maintained, and made publicly accessible in compliance with the most up-to-date FCOI regulations.
Definitions
-
Disclosure of significant financial interests means an Investigator’s disclosure of significant financial interests (SFIs) to an Institution.
-
Financial conflict of interest (FCOI) means a significant financial interest that could directly and significantly affect the design, conduct, or reporting of PHS-funded research.
-
FCOI report means an Institution’s report of a financial conflict of interest to a PHS Awarding Component.
-
Financial interest means anything of monetary value, whether the value is readily ascertainable.
-
HHS means the United States Department of Health and Human Services, and any components of the Department to which the authority involved may be delegated.
-
Institution means any domestic or foreign, public, or private, entity or organization (excluding a Federal agency) that is applying for, or that receives, PHS research funding.
-
Institutional responsibilities mean an Investigator’s professional responsibilities on behalf of the Institution, and as defined by the Institution in its policy on financial conflicts of interest, which may include for example: activities such as research, research consultation, teaching, professional practice, institutional committee memberships, and service on panels such as Institutional Review Boards or Data and Safety Monitoring Boards.
-
Investigator means the project director or principal Investigator and any other person, regardless of title or position, who is responsible for the design, conduct, or reporting of research funded by the PHS, or proposed for such funding, which may include, for example, collaborators or consultants.
-
Manage means taking action to address a financial conflict of interest, which can include reducing or eliminating the financial conflict of interest, to ensure, to the extent possible, that the design, conduct, and reporting of research will be free from bias.
-
PD/PI means a project director or principal Investigator of a PHS-funded research project; the PD/PI is included in the definitions of senior/key personnel and Investigator under this subpart.
-
PHS means the Public Health Service of the U.S. Department of Health and Human Services, and any components of the PHS to which the authority involved may be delegated, including the National Institutes of Health (NIH).
-
PHS Awarding Component means the organizational unit of the PHS that funds the research that is subject to this subpart.
-
Public Health Service Act or PHS Act means the statute codified at 42 U.S.C. 201 et seq.
-
Research means a systematic investigation, study or experiment designed to develop or contribute to generalizable knowledge relating broadly to public health, including behavioral and social-sciences research. The term encompasses basic and applied research (e.g., a published article, book, or book chapter) and product development (e.g., a diagnostic test, software, or drug). As used in this subpart, the term includes any such activity for which research funding is available from a PHS Awarding Component through a grant or cooperative agreement, whether authorized under the PHS Act or other statutory authority, such as a research grant, career development award, center grant, individual fellowship award, infrastructure award, institutional training grant, program project, or research resources award.
-
Senior/key personnel means the PD/PI and any other person identified as senior/key personnel by the Institution in the grant application, progress report, or any other report submitted to the PHS by the Institution under this subpart.
-
Significant financial interest means:
(1) A financial interest consisting of one or more of the following interests of the Investigator (and those of the Investigator’s spouse and dependent children) that reasonably appears to be related to the Investigator’s institutional responsibilities:
(a) With regard to any publicly traded entity, a significant financial interest exists if the value of any remuneration received from the entity in the twelve months preceding the disclosure and the value of any equity interest in the entity as of the date of disclosure, when aggregated, exceeds $5,000. For purposes of this definition, remuneration includes salary and any payment for services not otherwise identified as salary (e.g., consulting fees, honoraria, paid authorship); equity interest includes any stock, stock option, or other ownership interest, as determined through reference to public prices or other reasonable measures of fair market value;
(b) With regard to any non-publicly traded entity, a significant financial interest exists if the value of any remuneration received from the entity in the twelve months preceding the disclosure, when aggregated, exceeds $5,000, or when the Investigator (or the Investigator’s spouse or dependent children) holds any equity interest (e.g., stock, stock option, or other ownership interest); or
(c) Intellectual property rights and interests (e.g., patents, copyrights), upon receipt of income related to such rights and interests.
(2) Investigators also must disclose the occurrence of any reimbursed or sponsored travel (i.e., that which is paid on behalf of the Investigator and not reimbursed to the Investigator so that the exact monetary value may not be readily available), related to their institutional responsibilities; provided, however, that this disclosure requirement does not apply to travel that is reimbursed or sponsored by a Federal, state, or local government agency, an Institution of higher education as defined at 20 U.S.C. 1001(a), an academic teaching hospital, a medical center, or a research institute that is affiliated with an Institution of higher education. The Institution’s FCOI policy will specify the details of this disclosure, which will include, at a minimum, the purpose of the trip, the identity of the sponsor/organizer, the destination, and the duration. In accordance with the Institution’s FCOI policy, the institutional official(s) will determine if further information is needed, including a determination or disclosure of monetary value, in order to determine whether the travel constitutes an FCOI with the PHS-funded research.
(3) The term significant financial interest does NOT include the following types of financial interests: salary, royalties, or other remuneration paid by the Institution to the Investigator if the Investigator is currently employed or otherwise appointed by the Institution, including intellectual property rights assigned to the Institution and agreements to share in royalties related to such rights; any ownership interest in the Institution held by the Investigator, if the Institution is a commercial or for-profit organization; income from investment vehicles, such as mutual funds and retirement accounts, as long as the Investigator does not directly control the investment decisions made in these vehicles; income from seminars, lectures, or teaching engagements sponsored by a Federal, state, or local government agency, an Institution of higher education as defined at 20 U.S.C. 1001(a), an academic teaching hospital, a medical center, or a research institute that is affiliated with an Institution of higher education; or income from service on advisory committees or review panels for a Federal, state, or local government agency, an Institution of higher education as defined at 20 U.S.C. 1001(a), an academic teaching hospital, a medical center, or a research institute that is affiliated with an Institution of higher education.
-
Small Business Innovation Research (SBIR) Program means the extramural research program for small businesses that is established by the Awarding Components of the Public Health Service and certain other Federal agencies under Public Law 97-219, the Small Business Innovation Development Act, as amended. For purposes of this subpart, the term SBIR Program also includes the Small Business Technology Transfer (STTR) Program, which was established by Public Law 102-564.
Policy Instructions and Disclosure Procedures
-
Responsibilities of the Institution
(1) In compliance with PHS FCOI Regulations, OMICSCRAFT’s Signing Official (SO) will ensure that all Investigators are informed of and in compliance with this Policy regarding financial conflicts of interest, the Investigator’s responsibilities of disclosing SFI’s, and with PHS FCOI Regulations.
(2) As applicable, the SO will maintain records of all Investigator disclosures of financial interests and OMICSCRAFT’s review of, and response to, such disclosures under OMICSCRAFT’s Policy or retrospective review, regardless of whether a disclosure resulted in the OMICSCRAFT’s determination of FCOI: (a) For at least three years following the submission date of the final expenditures report or from the date of submission of the quarterly or annual financial report; or (b) From other dates stipulated by 45 C.F.R. 74.53(b) and 92.42(b).
(3) All records of Investigator disclosures of financial interests and OMICSCRAFT’s review of, and response to, such disclosures will be maintained: (a) In hard copy of the project binder specific to the PHS-funded work; and (b) Electronically, filed in the “FCOI” folder of the project file specific to the PHS-funded work on the server.
-
Policy Application to Sub-awardees, Subcontractors, and OMICSCRAFT’s Affiliates
Sub-awardees, subcontractors, and other OMICSCRAFT’s affiliates collaborating on PHS-funded research will be required to confirm that they have their own FCOI policy in place that conforms to PHS FCOI 2011 Regulations prior to a grant being funded. If they do not have their own FCOI policy, they will be required to comply with OMICSCRAFT’s FCOI Policy.
-
Mandatory Training Requirements
(1) Per PHS FCOI Regulations, all Investigators will be required to complete FCOI training on both Federal and Institutional policies. This training must be completed prior to engaging in research related to any PHS-funded grant and at least every four years, and immediately if any of the following circumstances apply: (a) The OMICSCRAFT FCOI Policy or procedures contained within or the PHS Financial Disclosure Form are revised in any manner that affects the requirements of Investigators; (b) An Investigator joins OMICSCRAFT; or (c) OMICSCRAFT is made aware of an Investigator who is not in compliance with this FCOI Policy or management plan.
(2) To fulfill the Institutional training requirement, all OMICSCRAFT Investigators will be required to read through this OMICSCRAFT FCOI Policy for PHS Funding and sign to attest to the fact that they have read and understood all policies, rules, and regulations contained within this document, and that they understand their responsibilities as an Investigator to disclose any and all significant financial interests.
(3) To fulfill the Federal training requirement, all OMICSCRAFT investigators must complete the following training program: “Financial Conflict of Interest Online Tutorial” (https://grants.nih.gov/grants/policy/coi/tutorial2018/story_html5.html). At the end of the tutorial, each person should fill out the Certificate of Completion and follow the instructions for documentation.
(4) Exceptions to these training requirements will be managed on a case-by-case basis as needed.
(5) All records of completion of training requirements will be maintained: (a) In hard copy, filed under the appropriate “FCOI Compliance Documentation” binder; and (b) Electronically, filed in the appropriate “Financial Conflict of Interest” folder.
-
Investigator Disclosure Requirements
(1) Per PHS FCOI Regulations, it is OMICSCRAFT’s Policy that all SFI’s be disclosed to OMICSCRAFT SO: (a) At the time of application for funding by each Investigator, including subrecipient Investigators as applicable, planning to be involved in PHS/NIH funded research; (b) Annually by each Investigator, including sub-recipient Investigators as applicable, involved with the award for the award period to update disclosures of SFIs; and (c) Within 30 days of an Investigator, including sub-recipient Investigators as applicable, discovering, or acquiring (e.g., through purchase, marriage, or inheritance) a new SFI.
(2) OMICSCRAFT will solicit and review disclosures of SFI(s) of all Investigators and their immediate family (including spouse/domestic partner and/or dependent children) related to the Investigator’s institutional responsibilities. All Investigators will utilize the “Financial Disclosure Form for Investigators in PHS Research” to either disclose SFIs or declare that there are no SFI’s.
Management of Financial Conflicts of Interest
-
Review of SFI disclosure
All disclosures of SFIs will be reviewed by OMICSCRAFT’s SO in a prompt manner such that within 60 days of receipt of the disclosure, the SFI can be assessed, a management plan developed, and all reviews and approvals necessary can be obtained. The SO will:
(1) Record and review all “Financial Disclosure for Investigators in PHS Research” forms from PHS-funded Investigators at OMICSCRAFT;
(2) Assess the nature of the SFI to determine whether the Investigator working on the PHS-funded research has a FCOI;
(3) Provide information concerning FCOI’s to the expenditure of PHS funds, which will be updated at least annually and within 60 days of changes to Statement of Financial Interest Disclosure forms, whichever occurs first.
-
Management of FCOI’s
The OMICSCRAFT SO will develop a management plan based on the nature of the FCOI and will monitor investigator compliance with the management plan on an ongoing basis until the completion of the PHS-funded research project. Examples of conditions or restrictions that could be established include, but are not limited, to:
(1) Public disclosure of FCOI’s;
(2) Appointment of independent monitor(s) who will be able to prevent the FCOI from biasing the design, conduct, and reporting of PHS-funded research;
(3) Modification of the research plan;
(4) Change of Investigator(s) or roles/responsibilities of Investigator, or exclusion of Investigator from participating in all or a portion of research;
(5) Reduction or elimination of the financial interest (e.g., sale of an equity interest); and
(6) Severance of relationships that create FCOI’s.
-
OMICSCRAFT Reporting Requirements
(1) Per PHS FCOI Regulations, OMICSCRAFT will submit to the NIH through the eRA Commons FCOI Module both initial and ongoing FCOI reports: (a) Prior to the expenditure of funds; (b) Within 60 days of identifying a new FCOI during the period of the award; and (c) Annually when grantee is required to submit the annual progress report, including multi-year progress report, or at time of extension, to report on the status of FCOI and any changes in the management plan.
(2) As per the PHS FCOI Regulations, FCOI reports will include: (a) Grant number; (b) PD/PI or contact PD/PI; (c) Name of Investigator with FCOI; (d) Name of entity with which Investigator has FCOI; (e) Nature of FCOI; (f) Value of the financial interest reported as a range ($0-4,999; $5,000-9,999; $10,000-19,999), in increments of $20,000 if between $20,000-100,000, in increments of $50,000 if above $100,000, or a statement that the value cannot be readily determined; (g) Description of how the SFI relates to PHS-funded research and basis for OMICSCRAFT’s determination that the SFI conflicts with this research; and (h) Key elements of OMICSCRAFT’s management plan.
-
Public availability of FCOI disclosure
As per the PHS FCOI Regulations, disclosure of SFIs that are still held by the Investigator for the PHS-funded research project, determined by OMICSCRAFT to be related to the PHS-funded research, and determined by OMICSCRAFT to be a FCOI disclosure of FCOI’s will be made available as a written response within five business days of a request. The information made available by OMICSCRAFT on in a written response will include the following information: (a) Name of Investigator with FCOI; (b) Title and role of the Investigator in the PHS-funded research project; (c) Name of entity with which Investigator has FCOI; (d) Nature of the FCOI; and (e) Value of the financial interest, reported as a range ($0-4,999; $5,000-9,999; $10,000-19,999), in increments of $20,000 if between $20,000-100,000, in increments of $50,000 if above $100,000, or a statement that the value cannot be readily determined through references to public prices or other reasonable measures of fair market value.
Enforcement of Policy and Noncompliance
-
All Investigators and relevant OMICSCRAFT personnel and collaborators are required to comply with this Policy in full. Violations of this policy may result in disciplinary or other appropriate action.
-
If an FCOI is not identified or managed in a timely manner (including Investigator failure to disclose SFI(s), OMICSCRAFT’s failure to review or manage FCOI(s), or failure to comply with the management plan), OMICSCRAFT will within 120 days of the determination of noncompliance notify the NIH and submit a retrospective review, as per PHS FCOI Regulations. The retrospective review will include the following information: (a) Grant number; (b) Grant title; (c) PD/PI or contact PD/PI; (d) Name of Investigator with FCOI; (e) Name of entity with which Investigator has FCOI; (f) Reason(s) for retrospective review; (g) Detailed methodology used for retrospective review (e.g., details of review process, composition of the review panel, documents reviewed); (h) Findings and conclusions of the review; and (i) If warranted by the retrospective review, update and revise the previously submitted FCOI report.
-
In the case that a retrospective review finds that Investigator non-compliance with this FCOI Policy, the PHS FCOI Regulations, or a management plan appears to have biased the design, conduct, or reporting of PHS-funded research, OMICSCRAFT will promptly submit a mitigation report to the NIH Awarding Component. The mitigation report will include: (a) Key elements documented in the retrospective review; (b) Description of the impact of bias on the research project; and (c) Management plan(s) to eliminate or mitigate the bias on the design, conduct, or report of research.